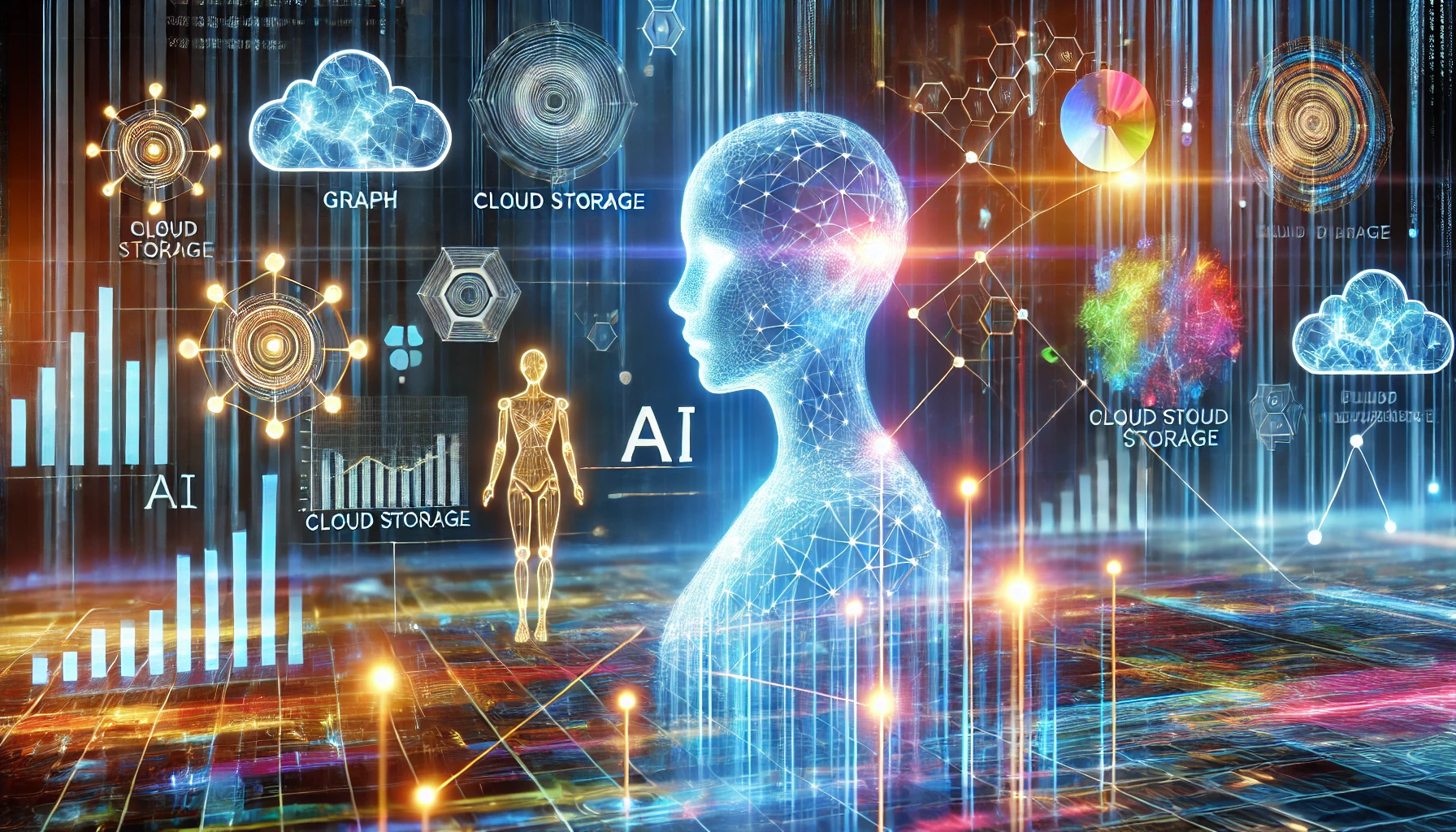
Unlocking the Power of Model Context Processors (MCP) for Google Analytics using Python
Model Context Processors (MCP) streamline AI workflows by efficiently managing data integration. Using FastMCP with Google Analytics simplifies API interactions, enhances automation, and improves maintainability. The provided Python example demonstrates how MCP extracts traffic data, optimizing AI-driven insights. MCP ensures structured, scalable, and reusable analytics solutions for businesses.
Why Use MCP?
As AI-driven applications become more sophisticated, managing and processing model context efficiently is crucial. Model Context Processors (MCP) provide an abstraction layer that allows developers to seamlessly integrate machine learning tools with various data sources, enabling more structured and efficient AI-driven workflows.
MCP, particularly when used with FastMCP, simplifies interaction with APIs, manages data transformations, and ensures that AI models operate with the most relevant contextual information. This is particularly useful for businesses analyzing user engagement and traffic patterns in Google Analytics.
Benefits of MCP
- Simplifies API Integrations: MCP provides a structured way to interact with external data sources, reducing boilerplate code.
- Enhances AI Workflows: By standardizing context management, MCP ensures AI models receive high-quality, structured inputs.
- Flexible & Scalable: MCP can be used across multiple analytics platforms and data processing tasks.
- Improves Maintainability: Using MCP minimizes hardcoded logic, making scripts more reusable and easier to maintain.
Example: Using MCP with Google Analytics
Below is a Python snippet demonstrating how to use FastMCP to fetch traffic data from Google Analytics via the official SDK:
1from google.analytics.data_v1beta import BetaAnalyticsDataClient
2from google.analytics.data_v1beta.types import RunReportRequest, DateRange, Metric, Dimension
3from mcp.server.fastmcp import FastMCP
4
5mcp = FastMCP("Google Analytics")
6
7# Load Google Analytics client
8GA_PROPERTY_ID = "xxxx"
9GA_CREDENTIALS = "/path/to/service_account.json"
10client = BetaAnalyticsDataClient.from_service_account_file(GA_CREDENTIALS)
11
12
13@mcp.tool()
14def get_traffic_data(start_date: str, end_date:str) -> list:
15 """
16 Return the number of visitors by traffic source, date and country.
17 """
18 request = RunReportRequest(
19 property=f"properties/{GA_PROPERTY_ID}",
20 dimensions=[
21 Dimension(name="date"),
22 Dimension(name="country"),
23 Dimension(name="sessionSource"),
24 Dimension(name="sessionMedium")
25 ],
26 metrics=[Metric(name="sessions"), Metric(name="activeUsers")],
27 date_ranges=[DateRange(start_date=start_date, end_date=end_date)],
28 )
29
30 response = client.run_report(request)
31
32 # Convert response to a structured list
33 results = []
34 for row in response.rows:
35 date = row.dimension_values[0].value
36 country = row.dimension_values[1].value
37 source = row.dimension_values[2].value
38 medium = row.dimension_values[3].value
39
40 sessions = int(row.metric_values[0].value)
41 users = int(row.metric_values[1].value)
42
43 results.append({
44 "date": date,
45 "country": country,
46 "source": source,
47 "medium": medium,
48 "sessions": sessions,
49 "users": users
50 })
51
52 return results
Understanding the Code
- Initializing MCP:
FastMCP("Google Analytics")
creates an instance of FastMCP tailored for Google Analytics data handling. - Google Analytics Client Setup: The script initializes a connection using a service account file.
- Fetching Metadata: The
get_all_metadata()
function retrieves available dimensions and metrics from Google Analytics. - Defining an MCP Tool: The
@mcp.tool()
decorator registersget_traffic_data
as an MCP tool, making it accessible within the AI workflow. - Running a Google Analytics Query: The
get_traffic_data
function requests traffic data segmented by date, country, source, and medium, then structures the response into a list.
Using with an LLM
MCP servers can be accessed from within Claude Desktop by installing your server implementation:
mcp install server.py
Now inside of Claude, you can query your Google Analytics data using natural language prompts:

What's useful here is that LLMs have built in knowledge graphs so-to-speak so you can ask for data about European countries, for example, without having to be explicit what is and isn't a European country.
Conclusion
MCPs significantly streamline the integration of AI-driven workflows with third-party APIs like Google Analytics. By structuring model context efficiently, MCP enhances automation, maintains clean code, and improves AI decision-making. Although they're not magic and still require some plumbing under the hood, MCPs provide a way to organize AI-native, agent centric software.
Be the first to experience Bashy
Bashy is currently in private beta, and we’re inviting innovative agencies to get early access. Sign up now to streamline your reporting and wow your clients.